Mozilla's Stance on User Data
Mozilla has long built its reputation on privacy, positioning Firefox as an alternative to data-hungry tech giants. For years, Firefox's messaging included explicit assurances that "Mozilla doesn't sell data about you, and we don't buy data about you." However, Yesterday (Feb 27, 2025), Mozilla updated its Data Privacy FAQ with more nuanced language, now stating: "Mozilla doesn't sell data about you (in the way that most people think about 'selling data'), and we don't buy data about you."
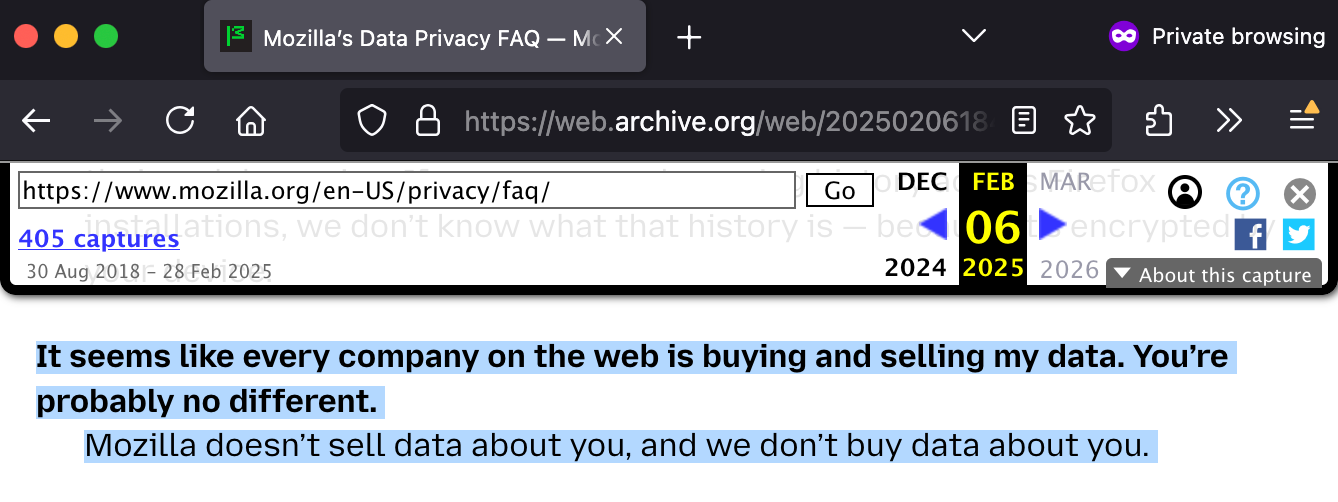
This rewording acknowledges that while Mozilla shares some data with partners for Firefox's "commercial viability," such data is stripped of identifying information, shared in aggregate, or protected via privacy-preserving techniques. The change was prompted by increasingly broad legal definitions of "data sales" in certain jurisdictions, making Mozilla cautious about making absolute promises.
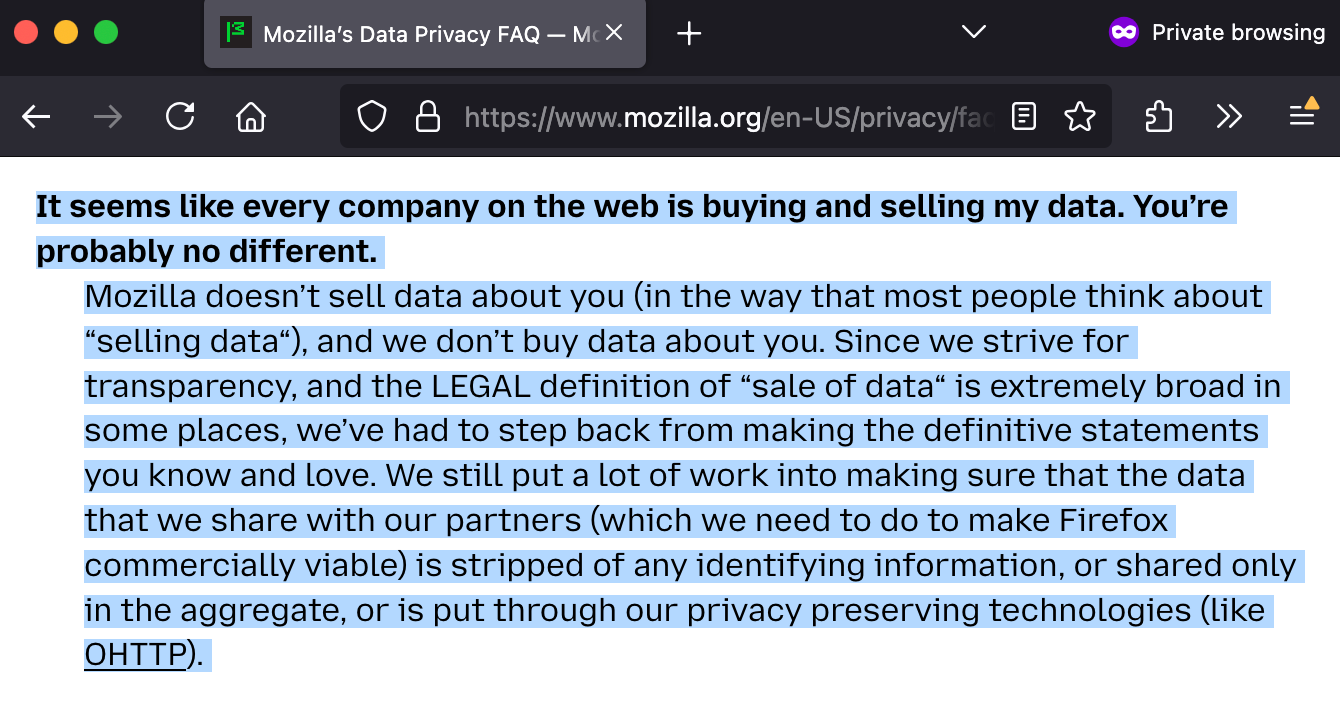
Mozilla maintains that its business model doesn't depend on selling personal data. The organization's primary revenue (over 90%) comes from search engine partnerships, particularly its agreement with Google to serve as Firefox's default search engine.
New Terms of Use and Privacy Policy Changes
27 February 2025, Mozilla introduced official Terms of Use for Firefox for the first time, along with an updated Privacy Notice. Previously, Firefox operated under an open-source license with informal privacy commitments. Mozilla explained this change as necessary to make its commitments "abundantly clear and accessible" in today's complex tech landscape.
The rollout sparked controversy among users when they noticed the removal of the explicit promise "Unlike other companies, we don't sell access to your data" from Mozilla's website and materials. This omission led to speculation that Mozilla might be preparing to sell user data, despite the organization's denials.
Another controversial point emerged from a clause in the new Terms of Use about user-submitted information. The terms asked users to "grant Mozilla a nonexclusive, royalty-free, worldwide license" to use information entered into Firefox. Taken at face value, this sounded as if Mozilla claimed rights over everything users type into the browser. Mozilla quickly clarified that this license only exists to make Firefox's basic functionality possible (processing URLs, performing searches, etc.) and that all data usage remains governed by the Privacy Notice's protections.
Community Reactions to Policy Changes
Many of these changes came to light before Mozilla's official announcements, thanks to its open development process. GitHub users spotted the changes in Mozilla's repositories, particularly the deletion of the line about not selling user data from the Firefox FAQ page.
Developers on GitHub expressed concern, with commenters urging that "the rationale for this ToS change is discussed in public," noting it seemed counter to Mozilla's principles of transparency and privacy.
On social media and forums, reactions ranged from disappointment to outrage. Some users accused Mozilla of betraying its privacy ethos, while others expressed skepticism about Mozilla's semantics—arguing there was little difference between "selling data" and "sharing it with partners" who provide revenue. Many long-time Firefox users discussed switching to alternative browsers like LibreWolf, Brave, or Safari.
Mozilla responded by publishing explanatory blog posts and engaging in forum discussions, but the initial lack of upfront communication allowed rumors to proliferate.
Privacy Incidents: Telemetry and Advertising Attribution
Beyond the Terms of Use controversy, Mozilla has faced other privacy-related challenges. In mid-2024, Mozilla implemented Privacy Preserving Attribution (PPA)—a system to help advertisers measure ad effectiveness without exposing individual user identities. However, it was enabled by default in Firefox 128, sending limited data about whether ads led to Firefox installations or website visits without explicit user opt-in.
This caught the attention of European privacy advocates. In September 2024, the Austrian digital rights group noyb filed a formal GDPR complaint, alleging that Mozilla had introduced tracking without users' consent. Mozilla defended PPA as privacy-preserving and less invasive than typical ad trackers, but admitted it "should have done more" to inform users and gather feedback.
A related issue involved Mozilla's use of Adjust, a third-party telemetry tool in mobile Firefox versions. In 2024, it came to light that Firefox for Android and iOS were sending data to Adjust to track how Mozilla's ads led to app installs, without prominent disclosure. Following community backlash, Mozilla removed the Adjust SDK from its mobile apps by August 2024.
Mozilla acknowledged regretting enabling such telemetry by default but explained the pressure it faced from advertisers who demand feedback on their campaigns. The compromise was to implement privacy-focused solutions and use aggregated metrics, though not everyone was convinced this was sufficiently transparent.
Product Updates Emphasizing Privacy
Despite these controversies, Mozilla continues to ship product updates aimed at enhancing user privacy. In Firefox version 135 (December 2024), Mozilla retired the old "Do Not Track" (DNT) setting in favor of the more robust Global Privacy Control (GPC) signal. Unlike DNT, which was widely ignored by websites, GPC has legal backing in regions like California, making it a more enforceable choice for users wanting to opt out of data collection.
Mozilla has also strengthened technological protections against tracking. In June 2022, Firefox rolled out Total Cookie Protection by default to all users, a milestone in browser privacy. This feature isolates cookies to the site where they were created, essentially giving each website its own "cookie jar" and preventing trackers from using third-party cookies to follow users across the web.
Additionally, Firefox's Enhanced Tracking Protection continues to block known trackers, fingerprinters, and cryptominers by default. Firefox's private browsing mode goes even further, blocking social media trackers and providing complete cookie isolation.
As Google Chrome moves to Manifest V3 (which limits the capabilities of ad-blockers), Mozilla has announced it will continue supporting the older Manifest V2 for Firefox add-ons alongside Manifest V3. This ensures users can keep using robust ad-blockers and privacy extensions without disruption, demonstrating Mozilla's willingness to diverge from Chrome in defense of user choice.
Organizational Decisions and Notable Developments
One significant controversy involved Mozilla's partnership with OneRep for its "Mozilla Monitor Plus" service, which helps users remove personal information from data broker websites. In March 2024, an investigative report revealed that OneRep's founder and CEO also owned numerous people-search and data broker sites—the very kind of privacy-invasive services OneRep claimed to protect users from.
Mozilla quickly announced it would terminate the partnership, stating that "the outside financial interests and activities of OneRep's CEO do not align with our values." However, as of early 2025, Mozilla was still in the process of disentangling from OneRep, explaining that finding a replacement service was taking longer than anticipated.
Beyond specific controversies, Mozilla has been diversifying its product portfolio to reduce reliance on the Firefox-Google search deal for revenue. New initiatives include Mozilla VPN and Mozilla.ai, a startup focused on ethical AI. The organization has also made difficult financial decisions, including layoffs in recent years, to maintain stability while continuing to advocate for an open, privacy-respecting web.
Conclusion and Implications
Claims that "Mozilla is selling user data" are not supported by evidence—Mozilla's policies emphasize that any data sharing happens in a privacy-conscious way. However, by removing its absolute "we never sell data" pledge and adding legal language about data licenses, Mozilla inadvertently created doubt among its loyal users.
The community reactions demonstrate that Mozilla's user base holds it to a higher standard than other browser makers. Every move that hints at dilution of privacy or transparency faces immediate scrutiny. This pressure keeps Mozilla aligned with its founding principles, as evidenced by its quick responses to clarify policies or reverse course on contentious features.
For users, two points are clear: Firefox remains one of the most privacy-friendly mainstream browsers, with features like Total Cookie Protection, tracker blocking, and powerful extension support. Unlike many tech companies, Mozilla does not monetize by profiling users or selling targeted ads based on browsing history. However, users must stay vigilant to ensure Mozilla maintains its privacy commitments.
From an industry perspective, Mozilla's handling of these issues could influence broader norms. When Firefox pushes privacy features like cookie isolation or GPC signals, it pressures competitors to offer similar protections. Mozilla is essentially testing whether a major software product can sustain itself without compromising user trust.
In summary, Mozilla is navigating complex legal, financial, and perceptual challenges regarding user data while striving to uphold its core ethos: "Internet for people, not profit." As long as Mozilla continues to engage with its community and prioritize privacy in tangible ways, Firefox will likely retain its position as the browser of choice for privacy-conscious users, and its developments will continue to influence the broader fight for online privacy.
https://ift.tt/YGe6pwi
https://ift.tt/i9dtMrF
https://images.unsplash.com/photo-1657885428171-0e164036a1f5?crop=entropy&cs=tinysrgb&fit=max&fm=jpg&ixid=M3wxMTc3M3wwfDF8c2VhcmNofDh8fGZpcmVmb3h8ZW58MHx8fHwxNzQwNzcxNDgyfDA&ixlib=rb-4.0.3&q=80&w=2000
https://guptadeepak.weebly.com/deepak-gupta/mozillas-data-practices-and-privacy-evolution-recent-developments