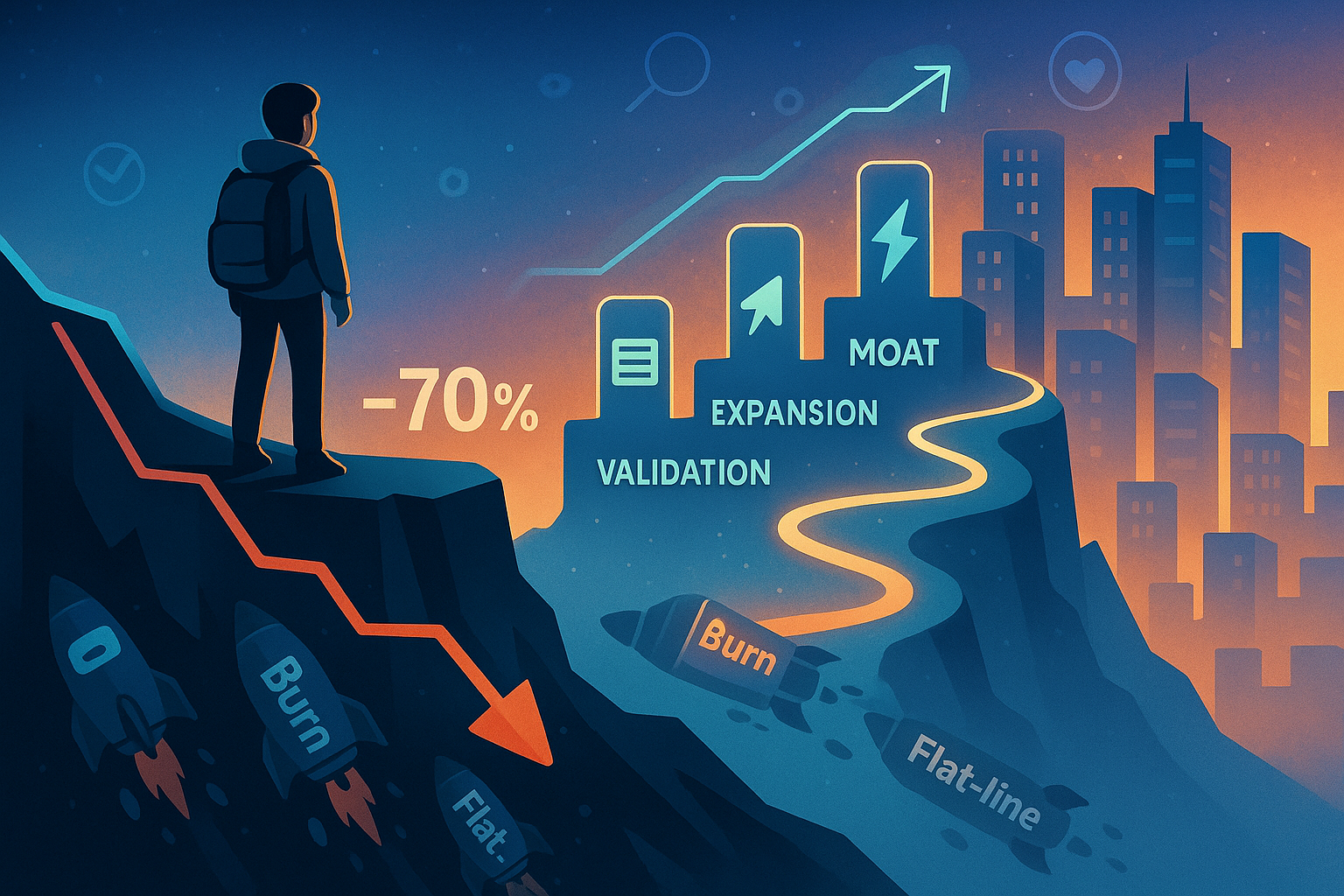
Here's the brutal truth: 90% of B2B SaaS companies fail because founders try to scale before they're ready, or they cling to tactics that worked at 10 customers when they have 100. If you're reading this, you probably know the feeling - that gnawing uncertainty about whether you should be hiring salespeople, launching that community, or finally implementing that pricing strategy you've been putting off.
The good news? The playbook exists. After analyzing recent case studies from companies like Notion, Figma, Linear, and dozens of emerging 2025 success stories, plus diving deep into founder psychology research, I've mapped the exact strategies that work at each critical growth stage. More importantly, I'll show you the mental shifts you need to make to avoid the psychological traps that kill most startups.
The three stages that matter
Every B2B SaaS company goes through three distinct growth phases, each requiring fundamentally different approaches:
- Stage 1 (1-10 customers): The validation phase - proving people will pay for your solution
- Stage 2 (10-100 customers): The pattern recognition phase - building repeatable growth systems
- Stage 3 (100-500 customers): The scaling phase - optimizing for market leadership
The companies that make it understand one critical principle: the tactics that got you here won't get you there. What works brilliantly at 10 customers can kill your growth at 100. Let me show you exactly what to do at each stage.
Stage 1: Your first 10 customers (the validation phase)
The psychological reality
75% of startup leaders experience imposter syndrome at this stage, and honestly, that's probably healthy. You should feel uncertain - you're literally trying to prove that your vision of the world is correct. The founders who fail here make the mistake of trying to act like they have 1,000 customers when they have 10.
The mindset shift you need: From product-focused to problem-obsessed. Stop thinking about scaling and start thinking about one thing - are you solving a real problem people will pay to fix?
What actually works
Founder-led everything: You need to personally handle every customer conversation, support ticket, and sales call. This isn't inefficient - it's essential data collection. Figma's founders spent three years building in stealth mode with essentially one full-time customer (Coda). They drove personally to debug issues. That obsessive customer focus created the foundation for their $10B+ valuation.
The "renaissance rep" approach: When you do hire your first salesperson (after 10-20 deals personally), hire someone who can handle the entire sales cycle. Always hire two reps initially - you need to A/B test approaches and create internal benchmarks. Look for product believers over pure sales experience.
Community building starts now: But focus on existing communities, not creating new ones. Notion built their early community by being active in productivity forums and maker spaces. They didn't launch "Notion Community" - they became valuable members of communities that already existed.
Metrics that matter
- Sean Ellis PMF Survey: Target >40% would be "very disappointed" without your product
- Net Promoter Score: Aim for >50
- Customer retention: >80% after 6 months
- Time to first value: Track how quickly new customers get their "aha moment"
The transition trigger
Move to Stage 2 when you have consistent month-over-month customer growth, proven ability to replicate success, and reduced dependency on founder involvement in every sale. Most importantly, you should have clear understanding of your ideal customer profile.
Stage 2: 10-100 customers (the pattern recognition phase)
The psychological shift
This is where most founders develop false confidence - they think they've "figured it out" because they have some customers. The real challenge is delegation anxiety. You built everything yourself, and now you need to trust others with your baby.
The key insight: Your job changes from doing everything to building systems that let others do things. This feels terrifying, but it's necessary.
Growth strategies that scale
Community-led growth becomes your secret weapon: This is when community building truly pays off. Notion's "Notion Pros" program turned power users into evangelists. They got early access to features, direct communication with the product team, and resources for community events. Result? Over 90% of their traffic came from organic search and social media.
The template strategy: Airtable built 200+ pre-built templates with SEO-optimized landing pages. Each template solved a specific job-to-be-done and created organic discovery. This approach helped them reach 250,000 enterprises and a $5.77B valuation. Templates accelerate activation and reduce time-to-value.
Content marketing systematization: Move from founder-led content to content systems. Create 2-3 educational posts per week, comprehensive guides, customer case studies, and video content. Focus on building a "content library" where each piece builds on previous topics. Budget 10-15% of ARR on content marketing.
Sales evolution
Product-led growth with sales assistance: Companies like Calendly nailed this model. They built intrinsic virality into the product (every shared link promoted the product) while layering in sales for larger deals. 66% of B2B leaders using channel sales expect >11% revenue jump in 2025.
When to add your VP of Sales: Only after you have 2+ successful reps hitting quota consistently. The VP should initially carry quota themselves and focus on building repeatable processes, not being a "sales magician."
Pricing evolution
Move from simple pricing to good-better-best tiered pricing. Most successful companies at this stage use three tiers:
- Basic: $49/month
- Professional: $99/month
- Enterprise: $199/month
Implement 10-20% annual price increases as you add value. Your customers expect it, and underpricing hurts more than it helps.
Customer success investment
Hire your first dedicated customer success manager after 25-30 customers. Budget 8-12% of ARR on customer success. Focus on onboarding optimization and churn prevention. Target net revenue retention of 100%+.
Critical metrics
- Monthly recurring revenue growth: Target 10%+ month-over-month
- Customer acquisition cost: $1,000-3,000 for B2B (varies by ACV)
- CAC payback period: <12 months
- Customer churn rate: <5% monthly
- Net revenue retention: >100%
Stage 3: 100-500 customers (the scaling phase)
The mental game changes
This is where burnout risk peaks - 53% of founders experience burnout at this stage. You're transitioning from "owner of everything" to "leader of leaders," and it feels like losing control. The psychological challenge is enormous.
The mindset shift: From owner to leader. You must move from controlling everything to empowering others. This requires what psychologists call "progressive responsibility transfer" - gradually handing off areas with clear success metrics.
Advanced growth strategies
Account-based marketing sophistication: Multi-touch ABM campaigns for enterprise prospects, personalized outreach sequences, account-based advertising. Use intent data and predictive analytics for prospecting. This is where your earlier community building pays massive dividends.
Product-led growth optimization: Advanced in-product analytics, viral loops, self-service onboarding optimization. Track product-qualified leads (PQLs) and create automated expansion revenue identification. Focus on expansion revenue - it should be 30%+ of total revenue.
Channel partnerships become viable: This is when partnerships truly pay off. Top SaaS companies see 58% of sales coming from partners. Look for reseller programs, referral partnerships, integration partnerships, and service provider partnerships.
The delegation framework
Type 1 vs Type 2 decisions (Bezos framework):
- Type 1: Irreversible, high-stakes (require deep founder consideration)
- Type 2: Reversible, low-risk (delegate and adjust)
Hire true owners: Find VPs who can own functional areas completely. Consider hiring a COO to handle 20-40% of operations. Build a management layer that can make decisions without you.
Advanced metrics
- Annual recurring revenue growth: 50-100%+ annually
- Net revenue retention: Target 110-125%
- Rule of 40: Growth rate + profit margin >40%
- LTV:CAC ratio: 3:1 to 5:1
- Customer lifetime value: $25K-100K+
Investment allocation
- Sales: 15-25% of ARR
- Marketing: 10-15% of ARR
- Customer Success: 8-12% of ARR
- R&D: 20-25% of ARR
The psychological survival guide
Common mental traps to avoid
The loyalty trap: 5-6 of your first 10 hires will remain at 50 employees, but only 2-3 will make it to 1,000. Don't keep people longer than their skills match company needs. This kills more scaling companies than any other single factor.
The hero complex: Believing you must solve every problem personally. This creates bottlenecks and prevents team development. Practice the "progressive responsibility transfer" - start with low-risk, high-learning delegation opportunities.
Perfectionism before scale: Stop over-engineering solutions before understanding true customer needs. Build for real problems, not imagined ones.
Mental health strategies that work
Build your support network immediately: Connect with other founders at your stage. Join groups or industry-specific communities. 72% of founders struggle with mental health issues - you're not alone, and peer support is proven to help.
Maintain customer contact: Don't lose touch with users as you grow. Schedule regular customer calls to stay connected to the problems you're solving.
Set real boundaries: Take actual vacations (2 weeks minimum per year), limit weekend work, and set a fair founder salary (average is $150K - don't underpay yourself).
Invest in professional help: Executive coaching, therapy, or advisory relationships. This isn't a luxury - it's essential infrastructure for scaling.
The 2025 market reality
What's changed
Longer sales cycles: Average B2B sales cycles increased 24% in 2025 due to economic uncertainty. Plan for 75+ day enterprise sales cycles.
Efficiency focus: The market rewards efficiency alongside growth. Track revenue per employee, not just growth rates. The new normal is 700 employees at $200M ARR ($300K revenue per employee).
AI integration opportunities: 71% of B2B buyers plan to invest in AI-powered software in 2025. Look for ways to integrate AI that actually improves user workflows, not just because it's trendy.
Community-led growth acceleration: Companies with dedicated community teams saw 22% growth rates vs. industry average of 19%.
Success patterns for 2025
Retention-first growth: Companies with 100%+ net revenue retention grow 1.8x faster than peers. Focus on expansion revenue over new customer acquisition after Stage 1.
Hybrid PLG models: Combine product-led growth with high-touch enterprise sales. The most successful 2025 companies use PLG for initial user acquisition and sales-assisted motion for expansion.
Community-driven content: User-generated content and community-driven product development create stronger moats than traditional marketing.
Your action plan
- Honest stage assessment: Which stage are you really in? Don't lie to yourself.
- Customer conversation audit: Are you still talking directly to customers weekly?
- Mental health check: When did you last take a real weekend off?
- Metric alignment: Are you tracking the right metrics for your stage?
Next 90 days
- Implement stage-appropriate systems: Don't build for future scale, build for current needs
- Start delegation experiments: Begin with low-risk, high-learning opportunities
- Build your founder support network: Find 2-3 peers at similar stages
- Customer success foundation: Implement retention processes if you haven't already
Long-term success habits
- Plan for psychological transitions: Each stage requires different mental frameworks
- Invest in leadership development: Your growth as a leader determines company growth
- Build sustainable practices: Systems that support long-term mental health
- Create legacy culture: Values that persist beyond founder involvement
The bottom line
Successful B2B SaaS scaling isn't about perfect execution - it's about executing the right tactics at the right stage. The companies that win understand that growth is as much psychological as it is operational. They invest in their mental health, build support networks, and adapt their strategies as they scale.
The data is clear: companies that systematically approach each growth stage with stage-appropriate strategies, while investing in founder psychological health, have a 3x higher chance of reaching $10M ARR. More importantly, their founders are still excited about running the business when they get there.
Your next move: Identify which stage you're really in, implement the core strategies for that stage, and start building the psychological foundation for the next one. The playbook exists - now it's time to execute it.
Remember: every successful B2B SaaS company started exactly where you are now. The difference is they understood that scaling is a game of stages, not just a race to grow faster.
https://bit.ly/4lehKVz
https://bit.ly/4l7aVEW
https://guptadeepak.com/content/images/2025/06/Founders-Survival-Guide-to-B2B-SaaS.png
https://guptadeepak.weebly.com/deepak-gupta/the-founders-survival-guide-to-b2b-saas-growth